Introduction – Sentiment Analysis
In the digital age, where data flows ceaselessly across social media, review platforms, and other online channels, understanding public sentiment has become a vital component for businesses, marketers, and researchers. Sentiment analysis, a branch of natural language processing (NLP), provides a means to quantify and interpret the emotions conveyed in textual data. This powerful tool allows organizations to gauge customer satisfaction, predict market trends, and even monitor brand reputation in real time.
However, with a multitude of AI tools available for sentiment analysis, choosing the right one can be overwhelming. Each tool comes with its own set of features, algorithms, strengths, and limitations. In this blog, we will explore the factors to consider when selecting an AI tool for sentiment analysis, delve into the different types of sentiment analysis, and review some of the top tools available in the market.
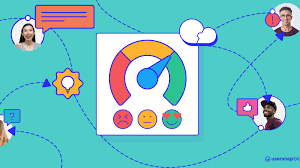
Understanding Sentiment Analysis
Before diving into the selection criteria, it’s essential to understand what sentiment analysis is and how it works. Sentiment analysis, also known as opinion mining, is the process of identifying and categorizing opinions expressed in a piece of text to determine the writer’s attitude toward a particular topic. This attitude could be positive, negative, or neutral.
Sentiment analysis can be applied to various forms of text, including:
- Customer reviews: To analyze how customers feel about a product or service.
- Social media posts: To gauge public opinion on trending topics, brands, or events.
- Surveys and feedback forms: To assess customer satisfaction and areas for improvement.
- News articles: To determine the tone of media coverage on specific issues or entities.
The analysis is typically conducted using machine learning algorithms, lexicon-based approaches, or a combination of both. Machine learning models are trained on large datasets to predict sentiment, while lexicon-based approaches use predefined lists of words associated with positive, negative, or neutral sentiments.
Types of Sentiment Analysis
Sentiment analysis is not a one-size-fits-all solution. Different types of sentiment analysis cater to various needs and applications. Understanding these types will help you determine which AI tool is best suited for your specific use case.
- Fine-grained Sentiment Analysis
- Description: This type of sentiment analysis goes beyond the basic positive, negative, or neutral classification. It provides more nuanced insights by categorizing sentiments into multiple levels, such as very positive, positive, neutral, negative, and very negative.
- Use Cases: Ideal for businesses that need detailed sentiment insights, such as understanding the intensity of customer feelings in product reviews or social media comments.
- Aspect-based Sentiment Analysis
- Description: Aspect-based sentiment analysis breaks down text into different aspects or features and assesses the sentiment associated with each one. For example, in a restaurant review, sentiments about food, service, and ambiance can be analyzed separately.
- Use Cases: Useful for companies looking to pinpoint specific areas of their products or services that need improvement or for identifying what aspects are most appreciated by customers.
- Emotion Detection
- Description: Emotion detection goes a step further by identifying specific emotions expressed in the text, such as joy, anger, sadness, or fear. This type of analysis often requires more sophisticated models, including deep learning algorithms.
- Use Cases: Particularly valuable in contexts where understanding the emotional undertone is crucial, such as customer support, mental health monitoring, or political campaign analysis.
- Intent Analysis
- Description: Intent analysis focuses on determining the intent behind a piece of text, such as whether a customer intends to purchase a product, seeks information, or wants to express dissatisfaction.
- Use Cases: Beneficial for e-commerce platforms, customer service automation, and targeted marketing campaigns where understanding customer intent can drive personalized engagement strategies.
- Multilingual Sentiment Analysis
- Description: This type of sentiment analysis deals with text in multiple languages. It’s especially important for global companies that receive feedback or engage with customers in different languages.
- Use Cases: Essential for businesses operating in international markets, allowing them to analyze sentiment across different regions and languages.
Factors to Consider When Choosing an AI Tool for Sentiment Analysis
Selecting the right AI tool for sentiment analysis requires careful consideration of various factors. Here’s a comprehensive guide to help you make an informed decision.
- Accuracy and Performance
- Importance: The accuracy of a sentiment analysis tool is paramount. An inaccurate tool can lead to misguided decisions and strategies. Performance, in terms of speed and efficiency, is also crucial, especially when analyzing large datasets.
- What to Look For:
- Check for accuracy metrics like precision, recall, and F1 score in the tool’s documentation or reviews.
- Consider tools that offer custom model training to improve accuracy based on your specific dataset.
- Evaluate the tool’s ability to handle large volumes of data without compromising speed.
Ease of Use and Integration
- Importance: A tool that is difficult to use or integrate with your existing systems can be a hindrance rather than a help. User-friendly interfaces and seamless integration capabilities can significantly enhance productivity.
- What to Look For:
- Look for tools with intuitive dashboards and clear documentation.
- Consider whether the tool offers APIs or plugins for easy integration with your CRM, social media platforms, or other data sources.
- Check for customization options that allow you to tailor the tool to your specific needs without requiring extensive technical knowledge.
- Language Support
- Importance: If your business operates in multiple regions, multilingual support is crucial. The ability to analyze sentiment across different languages ensures that you don’t miss out on valuable insights from non-English speaking customers.
- What to Look For:
- Ensure the tool supports the languages relevant to your business.
- Consider the tool’s ability to handle language nuances, such as slang, idioms, and cultural references, which can impact sentiment interpretation.
- Customization and Flexibility
- Importance: Different businesses have different needs. A one-size-fits-all approach may not work for everyone. Customization options allow you to tailor the sentiment analysis process to your specific requirements.
- What to Look For:
- Check if the tool allows for custom model training based on your dataset.
- Look for tools that offer flexibility in defining sentiment categories, especially if you need more granular insights.
- Evaluate the ability to add or modify lexicons to better suit your industry or domain.
- Scalability
- Importance: As your business grows, so will the volume of data you need to analyze. A scalable tool can handle increasing data loads without sacrificing performance.
- What to Look For:
- Assess the tool’s capacity to scale up as your data requirements grow.
- Consider whether the tool offers cloud-based solutions that can easily accommodate larger datasets.
- Check for options that allow you to add more users or integrate additional data sources as your needs evolve.
- Cost
- Importance: Budget constraints are a reality for most businesses. It’s important to choose a tool that offers good value for money while meeting your requirements.
- What to Look For:
- Compare pricing models—some tools charge per user, others per volume of data analyzed.
- Consider whether the tool offers a free trial or a freemium version to test its capabilities before committing.
- Evaluate the long-term cost implications, especially if you anticipate scaling up in the future.
- Support and Community
- Importance: Reliable customer support and a strong user community can be invaluable when you encounter issues or need guidance.
- What to Look For:
- Check if the tool offers 24/7 customer support, live chat, or a dedicated account manager.
- Explore the availability of tutorials, forums, and user communities where you can seek help and share knowledge.
- Consider tools with regular updates and active development, indicating a commitment to continuous improvement.
- Compliance and Data Security
- Importance: Data security is critical, especially when dealing with sensitive customer information. Compliance with data protection regulations like GDPR is also essential for businesses operating in certain regions.
- What to Look For:
- Ensure the tool complies with relevant data protection regulations, such as GDPR for European customers.
- Look for features like data encryption, secure APIs, and user access controls.
- Consider the tool’s data retention policies and whether it allows you to delete or anonymize data as needed.
Top AI Tools for Sentiment Analysis
With the criteria for selection in mind, let’s review some of the top AI tools for sentiment analysis currently available.
- MonkeyLearn
- Overview: MonkeyLearn is a no-code text analysis tool that offers sentiment analysis, among other NLP features. It’s known for its user-friendly interface and customizable models.
- Key Features:
- Custom model training with no coding required.
- Pre-built models for various sentiment analysis tasks.
- API integration for seamless connection with your existing systems.
- Support for multiple languages.
- Pros:
- Easy to use, even for non-technical users.
- High accuracy with the ability to train custom models.
- Flexible pricing plans.
- Cons:
- Limited to text data; may not be suitable for businesses needing multi-modal sentiment analysis.
- Some advanced features require a learning curve.
- Lexalytics
- Overview: Lexalytics specializes in text analytics and offers a comprehensive suite of tools for sentiment analysis, including emotion detection and aspect-based sentiment analysis.
- Key Features:
– Advanced sentiment analysis with support for emotion detection.- Ability to analyze text in 23 languages.
- On-premise and cloud deployment options.
- Customizable sentiment scoring and categorization.
- Pros:
- Robust and highly customizable.
- Excellent language support with a focus on nuance and context.
- Suitable for large-scale enterprise use.
- Cons:
- More complex to set up and use compared to no-code solutions.
- Higher cost, particularly for on-premise deployments.
- IBM Watson Natural Language Understanding
- Overview: IBM Watson’s Natural Language Understanding (NLU) is a powerful AI tool that provides deep insights into text data, including sentiment analysis, emotion detection, and keyword extraction.
- Key Features:
- Comprehensive text analysis capabilities beyond sentiment analysis.
- Customizable models with machine learning options.
- API access for easy integration with various platforms.
- Support for multiple languages and industry-specific models.
- Pros:
- Highly accurate and reliable, backed by IBM’s extensive research.
- Scalable and flexible, suitable for businesses of all sizes.
- Strong customer support and extensive documentation.
- Cons:
- Higher learning curve for non-technical users.
- Pricing can be prohibitive for small businesses or startups.
- Google Cloud Natural Language API
- Overview: Google Cloud’s Natural Language API offers sentiment analysis as part of its broader suite of text analysis tools. It leverages Google’s vast AI resources to provide fast and accurate sentiment analysis.
- Key Features:
- Sentiment analysis with support for entity recognition and syntax analysis.
- Pre-trained models with continuous improvement from Google’s AI research.
- API integration with Google Cloud services.
- Support for multiple languages.
- Pros:
- Fast and scalable, ideal for handling large datasets.
- Easy integration with other Google Cloud services.
- Competitive pricing, with a pay-as-you-go model.
- Cons:
- Limited customization options compared to other tools.
- Dependent on Google Cloud, which may not be suitable for all businesses.
- Microsoft Azure Text Analytics
- Overview: Microsoft Azure’s Text Analytics API offers a range of NLP features, including sentiment analysis, key phrase extraction, and language detection. It’s part of the broader Azure AI suite.
- Key Features:
- Sentiment analysis with multi-language support.
- Integration with other Azure services for comprehensive data analysis.
- Customizable models and scalable cloud infrastructure.
- Support for industry-specific applications.
- Pros:
- Seamless integration with Microsoft’s ecosystem.
- Scalable and flexible, suitable for enterprise-level use.
- Strong support and regular updates.
- Cons:
- Requires familiarity with Azure’s ecosystem, which can be complex.
- Higher cost for advanced features and large-scale deployments.
Conclusion
Choosing the right AI tool for sentiment analysis is a critical decision that can significantly impact your business’s ability to understand and respond to customer feedback, market trends, and brand perception. By considering factors such as accuracy, ease of use, language support, customization, scalability, cost, support, and data security, you can select a tool that not only meets your current needs but also grows with your business.
The tools discussed in this blog, including MonkeyLearn, Lexalytics, IBM Watson NLU, Google Cloud Natural Language API, and Microsoft Azure Text Analytics, are among the top options available. Each offers unique strengths and caters to different business requirements. Ultimately, the best tool for you will depend on your specific goals, resources, and the complexity of your sentiment analysis needs.
Investing in the right sentiment analysis tool can provide invaluable insights, enabling you to make data-driven decisions that enhance customer satisfaction, improve products and services, and strengthen your brand’s reputation in the market.
Add a Comment